
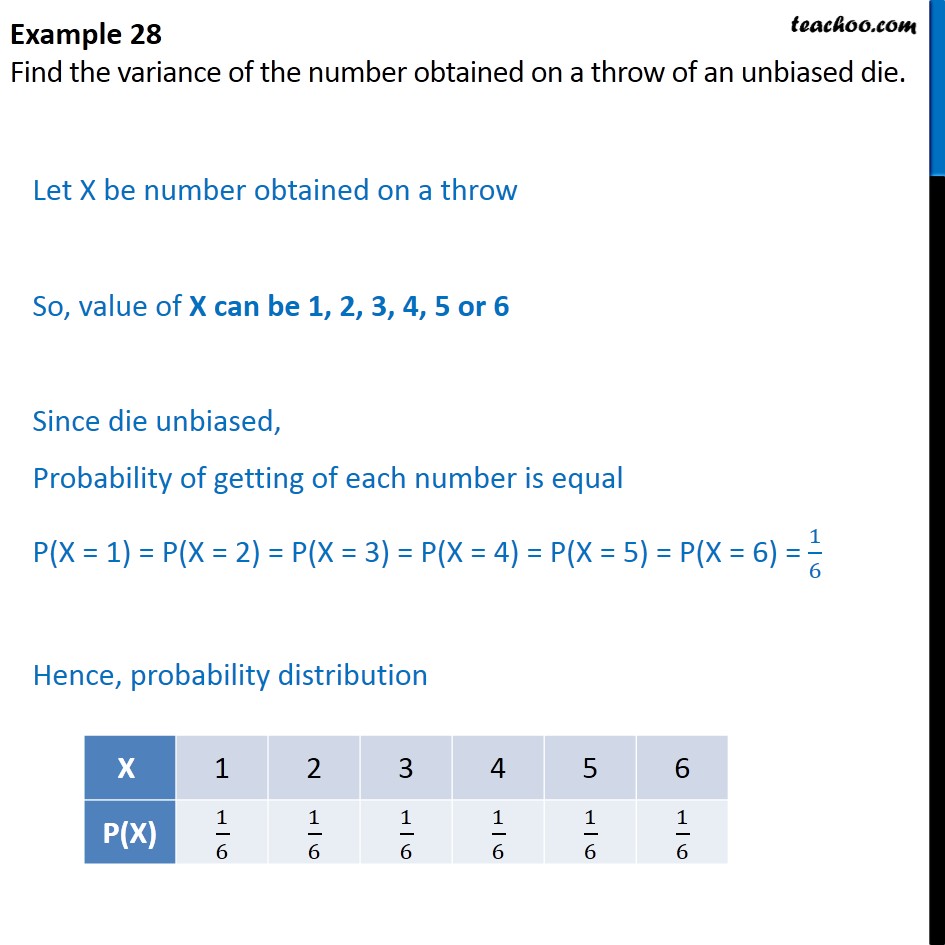
It may be that a collection of random variables is not independent even though all subcollections are. In general, if we have a collection of random variables X i, we say that they are all independent if the joint distribution is the product of the marginal distributions, i.e. Then Y and Z are independent, even though they are generated using the same physical process.
#Variance of coin flip mod
Roll one six-sided die X, and let Y = ⌈X/2⌉ and Z = X mod 2.This is an example of variables being independent because they are physically independent.
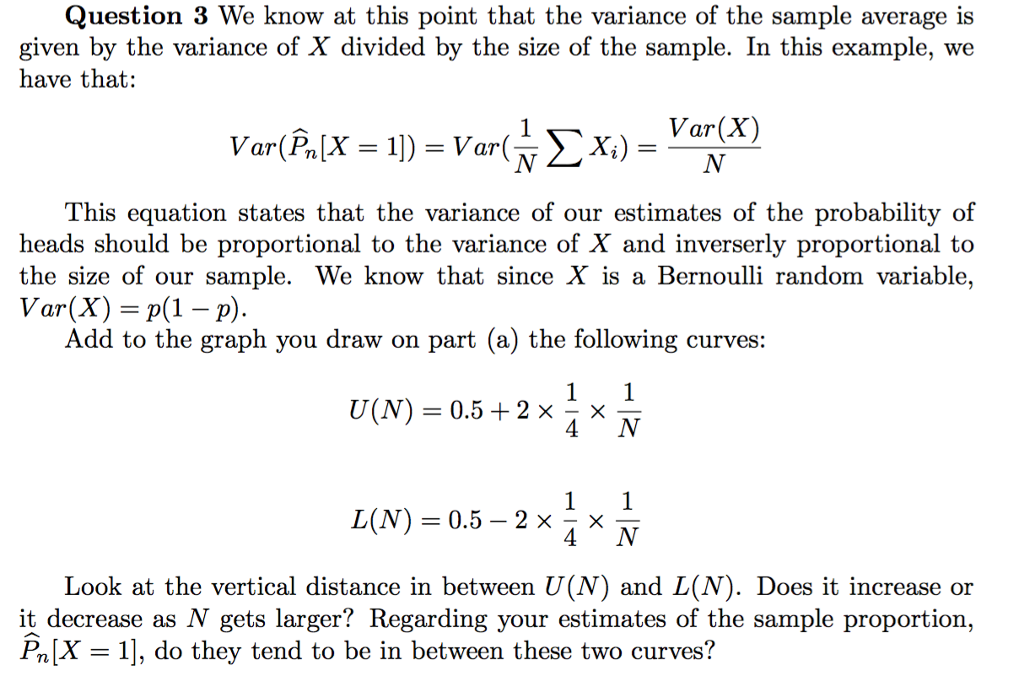
Since the sources are 42ms away from each other at the speed of light, we can assert that either X and Y are independent, or the world doesn't behave the way the physicists think it does. Place two radioactive sources on opposite sides of the Earth, and let X and Y be the number of radioactive decay events in each source during some 10ms interval.Now Z and X are not independent, because Pr = 0, which is not equal to Pr⋅Pr = (1/6)(1/36) = 1/216. Take the same X and Y, and let Z = X+Y.For example, a six-sided die corresponds to taking Ω = ] = (1/2)(1/2) = 1/4, which is a slightly easier computation than counting up the 9 cases (and then argue that each occurs with probability (1/6) 2, which requires knowing that X and Y are independent). The reason for this is we can just take Ω to be the range of the random variable, and define Pr for each ω∈Ω to be Pr. Often if we know the distribution of a random variable, we don't bother worrying about what the underlying probability space is. For discrete random variables (variables that take on only countably many values, each with nonzero probability), the distribution is most easily described by just giving the probability Pr for each value x. The distribution of a random variable describes the probability that it takes on various values. Then we can think of the entire sequence X as a sequence-valued random variable. , where X 0 = 0 and X n+1 is either X n+1 or X n-1, which being chosen by an independent fair coin flip. Or we could consider the infinite sequence X 0, X 1, X 2. Random sets and structures: Suppose that we have a set T of n elements, and we pick out a subset U by flipping an independent fair coin for each element to decide whether to include it. Then X is an integer-valued random variable. Counts: Flip a fair coin n times and let X be the number of times it comes up heads.Functions of random variables: Any function you are likely to run across of a random variable or random variables is a random variable, e.g.χ A) or using bracket notation (e.g.,, , ). Indicator variables are often written using the Greek letter chi (e.g. Indicator variables: The indicator variable for an event A is a variable X that is 1 if A occurs and 0 if it doesn't (i.e., X(ω) = 1 if ω∈A and 0 otherwise). This means that for each possible value x, there is an event with some probability of occurring that corresponds to X (the random variable, usually written as an upper-case letter) taking on the value x (some fixed value). Summary: effects of operations on expectation and variance of random variablesĪ random variable X is a variable that takes on particular values randomly.Application: lower bounds on random variables.Application: showing that a random variable is close to its expectation.Rewarding success and punishing failure.
